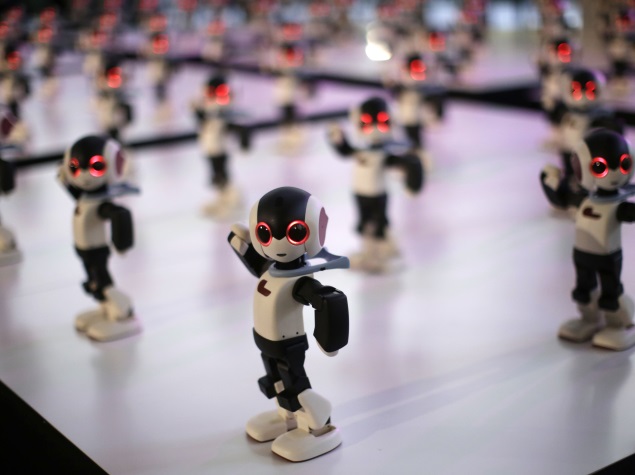
The problem researchers in robotics face is that robots do not intuitively ignore objects and actions that are irrelevant to the task at hand.
For example, if someone asked you to empty the trash can in the kitchen, you would know there is no need to turn on the oven or open the refrigerator. You would go right to the trash can.
Robots, however, lack that intuition. Most approaches to planning consider the entire set of possible objects and actions before deciding which course to pursue.
In other words, a robot might actually consider turning on the oven as part of its planning process for taking out the trash.
In complex environments, this leads to what computer scientists refer to as the “state-space explosion” – an array of choices so large that it boggles the robot mind.
“It’s a tough problem. We want robots having capabilities to do all kinds of different things, but then the space of possible actions becomes enormous,” said Stefanie Tellex, assistant professor of computer science at Brown.
“We don’t want to limit the robot’s capabilities, so we have to find ways to shrink the search space.”
The algorithm that Tellex and her students are developing does just that.
The algorithm augments standard robot planning algorithms using “goal-based action priors” – sets of objects and actions in a given space that are most likely to help an agent achieve a given goal.
The priors for a given task can be supplied by an expert operator, but they can also be learned by the algorithm itself through trial and error.
The researchers used the algorithm to have a robot help a person in the task of baking brownies. The algorithm was supplied with several action priors for the task.
For example, one action prior let the robot know that eggs often need to be beaten with a whisk. So when a carton of eggs appears in the robot’s workspace, it is able to anticipate the cook’s need for a whisk and hand him one.
In light of the results, Tellex says she sees goal-based action priors as a viable strategy to help robots cope with the complexities of unstructured environments – something that will be important as robots continue to move out of controlled settings and into our homes.
The work also shows the potential of virtual spaces like Minecraft in developing solutions for real-world robots and other artificial agents.
Minecraft is an open-ended game, where players gather resources and build all manner of structures by destroying or stacking 3D blocks in a virtual world.
“If you can do all of Minecraft you could solve anything. That’s pretty far off, but there are lots of interesting research objectives along the way,” Tellex said
[“source-gadgets.ndtv.com”]